Adjunct Professor
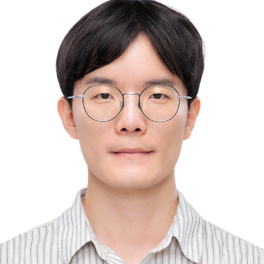
Sangho Lee
- Computational Cognitive Modeling
- Psychology-Based Artificial Intelligence
- Experimental Design Optimization
-
Education
The Ohio State University, Ph.D. in Psychology(2021)
-
Location
N4, Room No# 1311
- Phone
-
Laboratory
KAIST Psychological AI Lab
Biosketch
- Professor Sang Ho Lee is a cognitive scientist who combines insights from cognitive psychology with AI technologies to bridge theories of human cognition and AI methodologies. He is currently an assistant professor in the School of Digital Humanities and Computational Social Sciences at KAIST, and also serves as an adjunct professor in the Department of Brain and Cognitive Sciences.
- During his bachelor’s and master’s studies in psychology at Korea University, he developed a strong foundation in cognitive psychology and experimental methodology. He then earned a master’s degree in applied statistics and a Ph.D. in cognitive psychology at The Ohio State University, where he deepened his expertise in computational cognitive modeling and AI methodologies.
- Professor Sang Ho Lee aims to foster a virtuous cycle in which psychology and artificial intelligence continually inform and enhance one another. His research follows two main directions. The first, AI-based psychology, leverages AI models—now increasingly capable of functioning like humans—as cognitive models to explain human cognition, behavior, and brain activity. The second, psychology-based AI, aims to build AI models that emulate human information processing and behaviors by incorporating computational cognitive models grounded in cognitive psychology.
Key Papers
- Lee, S. H., Song, M. S., Oh, M. H., & Ahn, W. Y. (2024). Bridging the gap between self-report and behavioral laboratory measures: A real-time driving task with inverse reinforcement learning. Psychological Science, 35(4), 345-357.
- Lee, S. H., & Pitt, M. A. (2024). Implementation of an online spacing flanker task and evaluation of its test-retest reliability using measures of inhibitory control and the distribution of spatial attention. Behavior Research Methods, 1-12.
- Lee, S. H., Kim, D., Opfer, J. E., Pitt, M. A., & Myung, J. I. (2022). A number-line task with a Bayesian active learning algorithm provides insights into the development of non-symbolic number estimation. Psychonomic Bulletin & Review, 1-14.
- Lee, S. H., & Pitt, M. A. (2022). Individual differences in selective attention reveal the nonmonotonicity of visual spatial attention and its association with working memory capacity. Journal of experimental psychology. General, 151(4), 749-762.
Courses
- HSS333 Seminar in Psychology
- HSS322 Computational Cognitive Modeling Based on Psychological Data
- HSS552 Special Topics in Digital Humanities