Professor
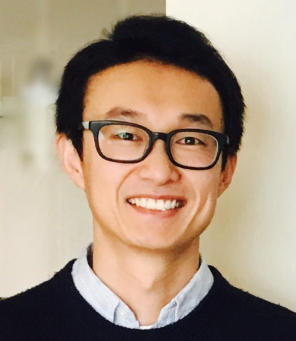
Sang Wan Lee
- Brain-inspired AI
- Decision neuroscience
- Computational neuroscience
- Reinforcement learning
-
Education
Ph D. in Electrical Engineering, KAIST (2009)
-
Location
W13, 501
- Phone
-
Laboratory
Laboratory for brain and machine intelligence
Biosketch
- I am currently an associate professor (tenured) in the Department of Brain cognitive sciences at KAIST, and an affiliated faculty of KAIST Institute for Health, Science, and Technology. I am also a founding director of the KAIST Center for Neuroscience-inspired Artificial Intelligence.
- I received my Ph.D. in Electrical Engineering and Computer Science from KAIST in 2009, working with Zeungnam Bien. I was a postdoctoral associate at MIT, working with Tomaso Poggio, followed by a Della Martin postdoctoral scholar at Caltech, working with John O'Doherty and Shinsuke Shimojo.
- I am the recipient of the Google Faculty Research Award (2016) and IBM Academic Awards (2021). I also won a few awards from KAIST, including KAIST Songam Distinguished Research Award (2019), KAIST Institute Faculty Award (2019), KAIST International Cooperation Award (2022), and KAIST Global Research Collaboration Award (2023). My lab was selected as a SW StarLab in AI (2023) by the Ministry of Science and ICT, Korea.
- My research focuses on understanding how the brain learns and makes inferences. To address this question, I have put together ideas from developing fields of machine learning and computational neuroscience. The approach is two-fold: 1) “Brain↦AI” aimed at understanding how the brain learns from a machine learning standpoint, and 2) “AI↦Brain” aimed at understanding why such neural processes occur.
Key Papers
- Lee J. H., Heo S Y., Lee S. W.*, Controlling human causal inference through in-silico task design. Cell Report, 2024.
- Kim, D., Jeong J., Lee S. W.*, Prefrontal solution to the bias-variance tradeoff during reinforcement learning. Cell Reports 37(13), 2021.
- Kim D, Park, G. Y., O′Doherty J. P.*, and Lee, S. W.*, Task complexity interacts with state-space uncertainty in the arbitration between model-based and model-free learning. Nature Communications 10, 5738, 2019.
- Lee, J. H.+, Seymour, B.+* Leibo, J. S., An, J., Lee, S. W.*, Towards high-performance, memory-efficient, and fast reinforcement learning: lessons from decision neuroscience. Science Robotics 4(26), eaav2975, 2019.
- G. Y. Park, J. Kim, B. Kim, S. W. Lee* and J. C. Ye* (co-corresponding), Energy-Based Cross Attention for Bayesian Context Update in Text-to-Image Diffusion Models. Neural Information Processing Systems (NeurIPS), 2023.
- Yaman A., Bredeche N., Caylak O., Leibo J., Lee S. W.* Meta-control of social learning strategies. PLOS Computational Biology, 2022.
- J. Shin, J. H. Lee, S. W. Lee*,In silico manipulation of human cortical computation underlying goal-directed learning. Neural Information Processing Systems (NeurIPS) Workshop on Human and Machine Decisions, 2021.
- G. Y. Park and S. W. Lee*, Reliably fast adversarial training via latent adversarial perturbation, Proceedings of International Conference on Computer Vision (ICCV), 2021. (oral; acceptance rate = 3%)
- Y-J. Cha, S. W. Lee*, Human uncertainty inference via deterministic ensemble neural networks. Proceedings of the 35th AAAI Conference on Artificial Intelligence (AAAI), 2021. (acceptance rate = 21%)
Courses
- How AI and the brain work
- Neuroscience-inspired Artificial intelligence